
FS-1119 | June 2020
What do the Numbers Really Mean? Interpreting Variety Trial Results
Land-grant universities across the United States conduct agricultural variety trials that provide farmers and other industry professionals with valuable data on crop performance. These data provide information regarding variety differences, such as yield, plant characteristics, disease resistance, and geographic performance, which aid producers in making the best variety selections for their farms. Trial data also provide industry professionals with an opportunity to evaluate novel variety performance through unbiased, third-party comparisons.
Reports from University variety trials are generated annually and can be lengthy, containing values, metrics, and other information that may require further explanation. If you plan to utilize variety trial data to make on-farm decisions, it is important to understand how to read and interpret the data so that you are able to draw the correct conclusions. It is easy to simply search the tables for the top-yielding variety at the location nearest to you and incorrectly dismiss the rest of the information.
Variety trial results and reports presented with statistical analyses provide a way for users to compare variety performance in a real-world setting through replicated plots. When using variety trial data, it is best to choose varieties with yield stability across geographical regions in Maryland and beyond. This may not be the highest yielding variety, but the variety with good yield across multiple locations and years. This variety is more likely to yield consistently across a wide range of environmental conditions.
This fact sheet explains how and why variety trials are implemented, walks a user through what the data mean, how to interpret the statistics, and draw sound conclusions based on those statistics.
Variety Trials Focus on Variety Performance
The primary objective of a variety trial field experiment is to test the performance of crop varieties relative to each other. To do this, the trials are designed to eliminate as much variability as possible to strengthen our ability to detect a difference in variety performance. Check varieties, or commercially-available varieties typically planted in the growing region, are included in variety trials to provide users of the trial results with a baseline for comparison. If farmers are familiar with the performance of a check variety on their farm or in their region, they will be able to better understand the performance of new or experimental varieties relative to the check.
As with any field trial, there will always be variability in the field that is difficult to control. Weather, soil type, and pest pressure are just a few of the factors that introduce variability into field research. To help control for this variability, variety trials are designed as small plots (often 10 feet wide x 30 feet long) and placed in a field with consistent soil types to minimize variability. The cover image of this fact sheet depicts a typical field plot variety trial. Each variety in the trial is replicated three to five times at random locations within the field. These replications can sometimes be blocked, or grouped together, to ensure all varieties are exposed to potential variability present in the field. Figure 1 depicts a randomized complete block plot design that contains four varieties replicated four times. Each of the four varieties is present once in each block. Every plot in the trial is treated identically in respect to planting date, planting depth, harvest date, data collection, pest management, fertility, etc. Variety is the only variable we allow to be different.
Example Field Plot Design
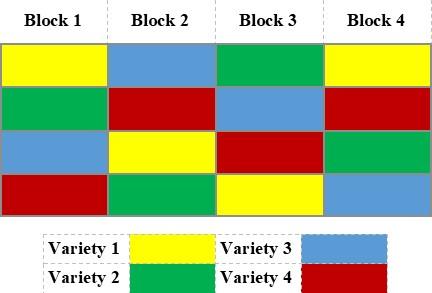
Data are Used to Compare Varieties
Table 1 is from the 2019 University of Maryland corn variety trials. The yield values (and other performance factors) presented in the table for each variety are an average of the yield for the three replicates of each variety planted and harvested at this location. The yield for LG62C02VTRIB, for example, reported as 223 bushels per acre in the table, is the average of 226, 231, and 212 bushels per acre, which were the yields collected for each of the three plots planted at this location. Since the yields were not identical for each of the plots, there is variation about the average yield. This variation is used to evaluate the difference in performance among the varieties.
The first step in comparing the varieties is to determine if there are any differences in the variable in question. For example, we want to know if there is a significant difference in yield between two corn varieties in a variety trial. To do this, an analysis of variance (ANOVA) statistical test is conducted on the yield data. The ANOVA test compares the variation in average yield for each variety to determine if the numerical difference in average yield is due to differences in variety performance or other unmeasured factors that introduce variability, such as soil type. The ANOVA test takes into account a confidence interval, which is defined prior to the study. In the scientific field of agricultural research, the confidence interval is usually set between 90-95%. This means that we are at least 90-95% confident that the differences observed between varieties is due to variety performance and not weather, soil type, or some other factor. This confidence means it is likely this difference in variety performance would be observed if the experiment was repeated under similar conditions.
Interpreting the Data to Help Make Planting Decisions
With the basic concept in mind, return to Table 1. One might think that hybrid DKC61-41RIB out-yielded NK 1205-3120 by 6.4 bushels per acre. It is true that numerically it did; however, we plan to utilize this data to make future decisions. We need to know if DKC61-41RIB will consistently out-yield NK 1205-3120, or if the experiment were repeated, would NK 1205-3120 be just as likely come out on top? This is where we use statistics to answer these questions.
You will find the statistical information to make inferences about the trial data in the bottom four rows of Table 1. The trial mean is the average of all varieties in the trial, which is an indicator of how the trial performed as a whole and is used to calculate the relative yield. Relative yield is simply the variety mean (average) yield divided by the overall trial mean, then multiplied by 100 to report as a percentage. The next two rows, Probability > F and LSD0.1, are generated from the ANOVA test and are critical to interpreting the data correctly.
Probability > F (sometimes indicated as P > F in other reports) indicates significant differences among varieties within performance metrics in a trial.
This value can be between 0 and 1, with a lower value indicating the likelihood of a true difference between variety performance. Researchers determine a cutoff value, typically either 0.05 or 0.1, before performing the analysis, and this cutoff value is related to the confidence intervals. If the P > F value is greater than the defined cutoff, then we cannot conclude that there were any yield differences between varieties in this trial, and if we repeated the experiment, we would not likely observe similar results. However, if the value is less than the defined cutoff, then there are yield differences between varieties that were caused by variety performance and, these results would likely be generated if the experiment were repeated. In this example, the confidence interval is 90%, therefore the cutoff for the P > F value is 0.1. If the confidence interval was set at 95%, the cutoff for P > F would be 0.05.
In the example in Table 1, there are significant differences in yield, moisture, and test weight due to variety, as these performance metrics have a P > F value less than 0.1. Since P > F is greater than 0.1 for lodging and plant population, we cannot conclude there are any differences in lodging or plant population as a result of variety. In other words, there is no difference in DKC59-82RIB with a lodging score of 1.4%, and P1197 AM, with a lodging score of 0%.
LSD₀.₁ or “least significant difference,” is the threshold that must be overcome to conclude that the performance of two varieties is significantly different.
Where the P > F value is below our pre-determined threshold, we have determined there is a difference in variety performance. The ANOVA test does not tell us which treatments are different, just that at least one difference exists. To determine which varieties are different, we need to refer to the LSD value. The LSD, or least significant difference, indicates the difference that two varieties must overcome to be considered “different” from each other. You will see this denoted as LSD₀.₁ in the table. In Table 1, performance metrics with P > F values below 0.1 will have a LSD₀.₁ value present, where metrics with P > F values above 0.1 do not. Specifically, for variety trial data, varieties are often compared to the highest yielding variety in the test (highlighted in blue in Table 1). For yield in Table 1, the LSD₀.₁ value is 12.4, meaning varieties must yield below 229.8 (or 12.4 bushels less than the highest yielding variety) to conclude that a variety will consistently yield less than the top yielding variety.
What are the take-away messages from Table 1 regarding variety yield performance? The top yielding variety in this trial was DKC59-82RIB. This variety yielded significantly more than all other varieties, except for SCS 1105AM, because the difference in yield between these two hybrids (8.4 bushels) does not exceed the LSD₀.₁ value of 12.4 bushels; therefore, they are not significantly different than each other. In Table 1, the lowest yielding variety (LCX10-98 VIP3110) did not yield significantly less than any other variety except for the top two (DKC59-82RIB and SCS 1105AM). It is important to note that LSD values will change depending on the dataset; so for one trial a significant difference may be 12.4 bushels whereas a different trial may only have an LSD₀.₁ of 4.6 bushels, for example. This is clearly seen when comparing the LSD₀.₁ values in the multiple tables in the 2019 University of Maryland Corn Hybrid Trials report (Fiorellino and Thorne, 2019).
The final statistic of interest is the coefficient of variation (CV%) which measures the variation in the data.
The CV (coefficient of variation) value indicates the amount of variation around the overall trial mean. The smaller the CV value, the less variability at this trial site. High CV values could be due to variability present in the field that was unaccounted for during trial design; for example, a difference in soil moisture affecting only part of the field. Other uncontrolled variability, such as weather conditions throughout the season, could also increase a trial’s CV value. In general, more variation in the dataset will require a larger LSD to separate differences between treatments.
Relative Yield, an important metric for decision making
The selection of a variety based solely on performance at one location is not recommended. When possible, it is recommended to select varieties based upon performance over a number of locations and years. In order to compare the performance of each variety across multiple trial locations, relative yield is calculated. Relative yield is the ratio of the yield of a variety to the mean yield of all the varieties at that location, expressed in percentage. A variety that has a relative yield consistently greater than 100 across all testing locations is considered to have excellent stability and will yield well across different geographic regions and potential weather conditions. On the UMD variety trials factsheets (found online at https://psla.umd.edu/extension/md-crops), the comparison of variety relative yield is found at the end of the reports.
Brand/Company | Hybrid Name¹ | Yield (bu/ac)² | Relative Yield | Moisture % | Lodging³ % | Test Weight (lb/bu)² | Population (plants/ac) |
---|---|---|---|---|---|---|---|
Dekalb |
DKC59-82RIB |
242.1* |
108.2 |
17.5 |
1.4 |
54.9 |
26680 |
Dekalb | DKC60-88RIB | 219.3 | 98.0 | 16.4 | 1.3 | 57.9 | 26680 |
Dekalb | DKC61-41RIB | 223.8 | 100.0 | 17.1 | 0 | 54.4 | 25410 |
Dekalb⁴ | DKC62-53RIB | 229.1 | 102.4 | 18.6 | 0 | 55.0 | 26342 |
Hubner | H4692RC2P | 223.0 | 99.6 | 18.1 | 0 | 54.9 | 25591 |
Local Seed Co. | LC0978 VT2PRIB | 216.9 | 96.9 | 16.8 | 2.3 | 57.6 | 27328 |
Local Seed Co. | LC1289 VT2PRIB | 219.1 | 97.9 | 17.2 | 0.7 | 56.1 | 25591 |
Local Seed Co. | LC1098 3330EZ | 216.5 | 96.7 | 19.5 | 0.6 | 51.8 | 29403 |
Dyna-Gro | D52VC63 | 222.4 | 99.4 | 17.4 | 0.7 | 56.0 | 26780 |
Syngenta/NK | NK1205-3120 | 217.4 | 97.1 | 19.4 | 0 | 53.8 | 26680 |
LG Seeds | LG5590VT2RIB | 224.4 | 100.3 | 18.2 | 1.3 | 54.5 | 27951 |
LG Seeds | LG62C02VT2RIB | 223.2 | 99.7 | 18.0 | 0.7 | 55.9 | 26136 |
Seed Consultants | SCS 1105AM | 233.7* | 104.4 | 17.0 | 0 | 57.1 | 26499 |
Pioneer⁴ | P1197 AM | 224.3 | 100.2 | 18.4 | 0 | 55.4 | 26136 |
Trial Mean | 223.8 | 100 | 17.8 | 0.6 | 55.4 | 26640 | |
Probability > F | 0.0805 | 0.0002 | 0.3777 | <0.0001 | 0.1504 | ||
LSD₀.₁ | 12.4 | 1.0 | NS⁵ | 1.0 | NS⁵ | ||
CV% | 4.5 | 6.2 | 187 | 3.1 | 6.1 |
- ¹See Table 7 (Fiorellino and Thorne, 2019) for trait designations for mid-season hybrids.
- ²Yields and test weights are reported at 15% moisture content.
- ³Lodging is recorded as percentage of plants that are broken below the ear and/or leaning 45° or greater.
- ⁴Hybrids in bold are checks.
- ⁵NS indicates that no statistically significant difference was observed for this characteristic.
- *Hybrids with an asterisk next to yield are not statistically different (Probability > F ≤ 0.1) compared to the top yielding hybrid (red text) at this location.
Use Statistics to Make Sound Conclusions from the Data
Understanding the importance of statistical significance will help you draw better conclusions from replicated variety trials. You will also find similar statistical methods in other types of replicated field research. These statistical analyses provide you with assurance that the conclusions are due to treatment effects (i.e. variety performance) and similar results are expected if the comparison was repeated under similar conditions. If you encounter data or reports that do not include any type of statistical analyses, it is important to realize that you cannot draw any conclusions about future performance or results from that dataset.
References and Suggested Reading
- Fiorellino, N. and L. Thorne. (2019). 2019 Maryland Corn Hybrid Performance Test. University of Maryland Agronomy Facts No. 54. https://cpb-us-e1.wpmucdn.com/blog.umd.edu/dist/a/434/files/2019/11/2019-Corn-Hybrid-Trials-Results.pdf
- University of Maryland Variety Trials. https://psla.umd.edu/extension/md-crops
ANDREW A. KNESS
akness@umd.edu
NICOLE M. FIORELLINO
nfiorell@umd.edu
This publication, What do the Numbers Really Mean? Interpreting Variety Trial Results (FS-1119) is a part of a collection produced by the University of Maryland Extension within the College of Agriculture and Natural Resources.
The information presented has met UME peer-review standards, including internal and external technical review. For help accessing this or any UME publication contact: itaccessibility@umd.edu
For more information on this and other topics, visit the University of Maryland Extension website at extension.umd.edu
University programs, activities, and facilities are available to all without regard to race, color, sex, gender identity or expression, sexual orientation, marital status, age, national origin, political affiliation, physical or mental disability, religion, protected veteran status, genetic information, personal appearance, or any other legally protected class.